
Returns : self estimator instanceĮstimator instance. Parameters : **params dictĮstimator parameters.
SKLEARN LDA COHERENCE SCORE UPDATE
Possible to update each component of a nested object. The method works on simple estimators as well as on nested objects None: Transform configuration is unchangedĮstimator instance. "default": Default output format of a transformer Parameters : X, default=NoneĬonfigure output of transform and fit_transform. When learning_method is ‘online’, use mini-batch update. Transform data X according to the fitted model. Get output feature names for transformation.Ĭalculate approximate perplexity for data X.Ĭalculate approximate log-likelihood as score. Learn model for the data X with variational Bayes method. fit ( X ) LatentDirichletAllocation(.) > # get topics for some given samples: > lda. What is the formula for cv coherence Ask Question Asked 4 years, 2 months ago Modified 5 months ago Viewed 13k times 2 I've recently been playing around with Gensim LDAModel. > X, _ = make_multilabel_classification ( random_state = 0 ) > lda = LatentDirichletAllocation ( n_components = 5. > from composition import LatentDirichletAllocation > from sklearn.datasets import make_multilabel_classification > # This produces a feature matrix of token counts, similar to what > # CountVectorizer would produce on text. exp_dirichlet_component_ ndarray of shape (n_components, n_features)Įxponential value of expectation of log topic word distribution. It can also be viewed as distribution over the words for each topic Number of times word j was assigned to topic i. Since the completeĬonditional for topic word distribution is a Dirichlet,Ĭomponents_ can be viewed as pseudocount that represents the Variational parameters for topic word distribution.

Attributes : components_ ndarray of shape (n_components, n_features) Pass an int for reproducible results across multiple function calls. random_state int, RandomState instance or None, default=None None means 1 unless in a joblib.parallel_backend context. Max number of iterations for updating document topic distribution in LDA states that each document in a corpus is a combination of a fixed number of topics. Stopping tolerance for updating document topic distribution in E-step. This post specifically focuses on Latent Dirichlet Allocation (LDA), which was a technique proposed in 2000 for population genetics and re-discovered independently by ML-hero Andrew Ng et al. Only used whenĮvaluate_every is greater than 0. In training process, but it will also increase total training time.Įvaluating perplexity in every iteration might increase training time Evaluating perplexity can help you check convergence Set it to 0 or negative number to not evaluate perplexity in Number of documents to use in each EM iteration. It only impacts the behavior in the fit method, and not the The maximum number of passes over the training data (aka epochs).
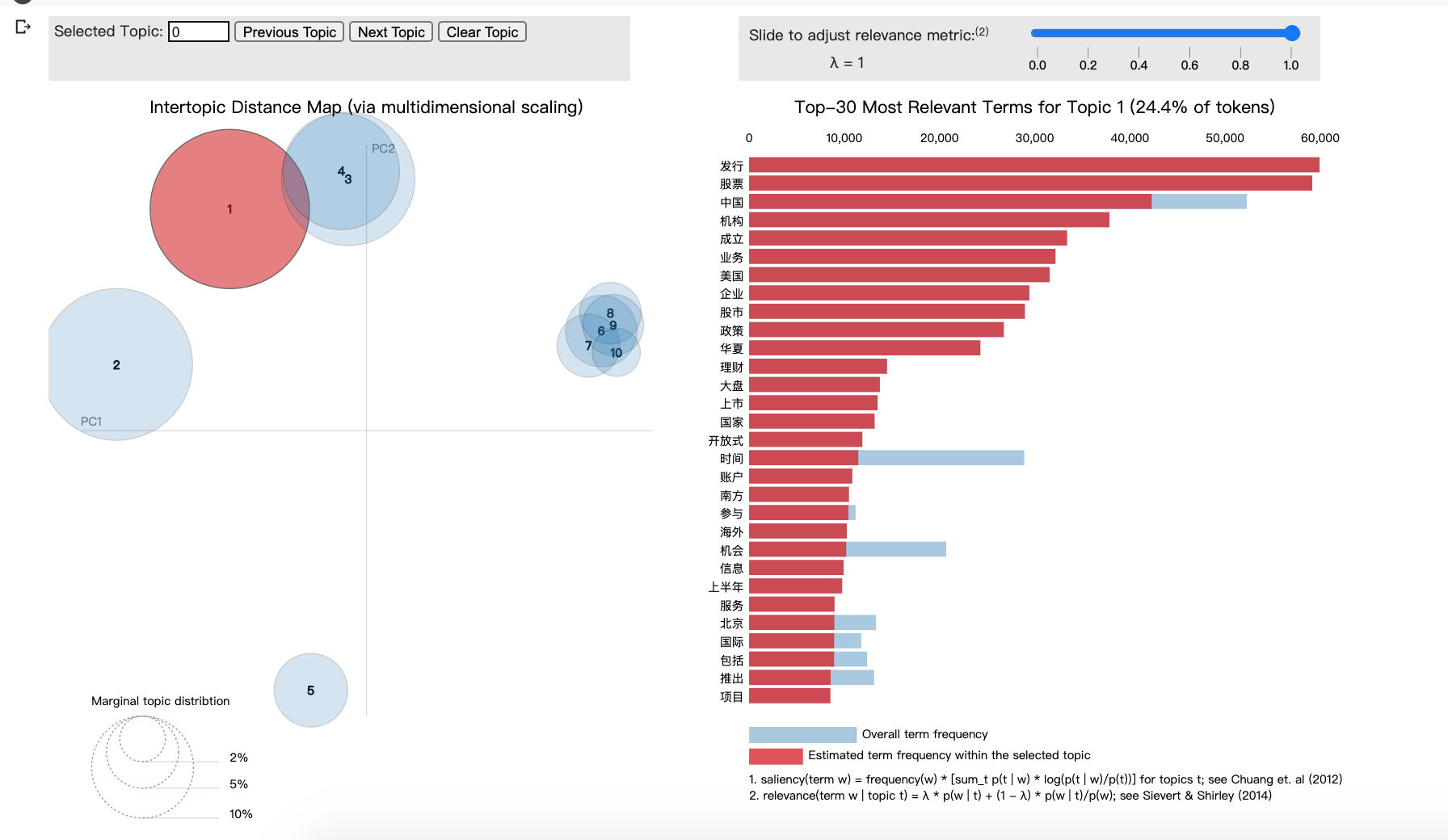
learning_offset float, default=10.0Ī (positive) parameter that downweights early iterations in online N_samples, the update method is same as batch learning. The value should be set between (0.5, 1.0] to guaranteeĪsymptotic convergence. It is a parameter that control learning rate in the online learning Changed in version 0.20: The default learning method is now "batch".
